Voronoi Polygons
What is Voronoi Polygons and When would you use Voronoi Polygons?
What is Voronoi Polygons?
Voronoi polygons are a spatial analysis concept used to divide a plane into regions based on proximity to a specified set of points. Each point is associated with a corresponding polygon or cell, and any location within a particular polygon is closer to its associated point than to any other. This spatial partitioning creates a clear boundary delineating areas of influence around each point.
Voronoi polygons can be created in Atlas by selecting a dataset containing points, choosing the Voronoi Polygons analysis from the data actions menu, and specifying any required parameters before running the analysis.
When would you use Voronoi Polygons?
Voronoi polygons are useful when you want to analyze spatial relationships and proximity between points within a dataset. This analysis can be applied in various fields such as urban planning, ecology, telecommunications, and resource allocation. By determining areas of influence or service areas around facilities, businesses can optimize location-based strategies, such as distribution points, healthcare services, and emergency response planning. It can also identify the nearest point of interest for any location within the defined spatial extent, enhancing decision-making processes based on spatial distribution.
Work faster with spatial data
Easily import data, automate analysis and build spatial apps for the web, all within a single software.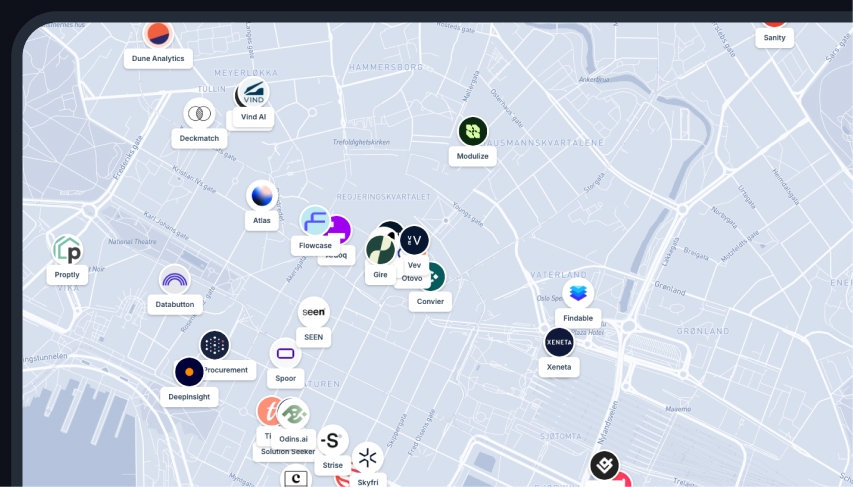
FAQs
How accurate are Voronoi polygons in representing real-world proximity?
Voronoi polygons provide mathematically precise boundaries based on the input points. However, real-world factors such as transportation networks and physical barriers might not be accounted for. For detailed applications, consider integrating additional spatial data.
Can Voronoi polygons overlap?
By definition, Voronoi polygons do not overlap. Each boundary is equidistant to two or more data points, ensuring discrete non-overlapping regions around each point.
What happens if there are duplicate points in my dataset?
If duplicate points exist in the dataset, each duplicate contributes to the same Voronoi polygon. You may want to clean the data to ensure accurate and meaningful analysis results.