Statistical Surface Modeling
Definition
Statistical surface modeling in the context of geographic information systems (GIS) refers to the process of using statistical methods to generate and analyze continuous surface data representations of spatial phenomena. These surfaces are created from discrete data points collected from various sources, such as satellite imagery, remote sensors, or field surveys. The goal is often to interpret spatial patterns, predict future values, or interpolate unknown values over a certain geographic area.
What is Statistical Surface Modeling?
Statistical surface modeling is a technique used to create continuous surface representations from scattered data points. This technique can be applied to a wide array of fields, including environmental science, urban planning, agriculture, and more. By applying different statistical methods such as interpolation, kriging, or spline modeling, one can estimate values at unknown points across a given space.
The process of statistical surface modeling typically involves several steps. Firstly, data collection is done through sampling or remote sensing methods. The collected data are then input into a GIS software that conducts spatial analysis. Different statistical methods are applied to this data to fit a surface model over the area of interest. This model helps predict or visualize spatial phenomena, trends, and relationships that are otherwise invisible in the raw data.
Statistical surface modeling is crucial for making informed decisions in various professional domains. For instance, in agriculture, it can help forecast crop yields by modeling soil properties across a field. In urban development, it can predict urban growth patterns, enabling better planning and resource allocation. Environmental scientists might use it to model climate variables, such as temperature or precipitation, for better understanding of weather patterns.
Work faster with spatial data
Easily import data, automate analysis and build spatial apps for the web, all within a single software.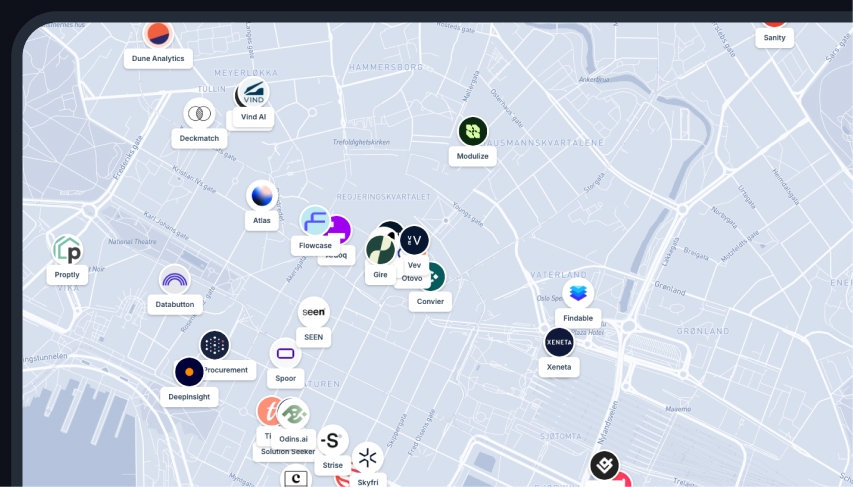
FAQs
What are some common methods used in statistical surface modeling?
Common methods used include interpolation techniques like Inverse Distance Weighting (IDW), kriging, and spline interpolation. Each method has its own advantages and is chosen based on the characteristics of the data and the specific requirements of the analysis.
What types of data can be used in statistical surface modeling?
Data types typically used include point data derived from samples, lines representing features such as roads or river networks, or polygon data representing areas such as land use zones. These datasets can originate from field surveys, satellite imagery, or sensor networks.
Why is statistical surface modeling important in GIS?
Statistical surface modeling is important because it transforms discrete data into continuous data, allowing for a more comprehensive analysis of spatial patterns and trends. This is essential for decision-making processes in resource management, urban planning, environmental protection, and other fields reliant on spatial data.
How does kriging differ from other interpolation methods?
Kriging is a geostatistical interpolation method that not only predicts unknown values but also provides a measure of uncertainty for these predictions. This is achieved by considering both the distance and degree of variation among the collected data points, making kriging particularly powerful for spatial precision.
Can statistical surface modeling be used for real-time data analysis?
Yes, statistical surface modeling can be adapted for real-time data analysis, especially with advances in GIS software and computational power. However, the accuracy of real-time models depends on the frequency and quality of the incoming data.