Spatial Statistics
Definition
Spatial statistics, as the name suggests, is the field of statistics dedicated to spatial data or data associated with locations. This involves any data that can be represented in some spatial form such as map points, polygons, lines, or pixels in an image.
What is Spatial Statistics?
Spatial statistics is an area of statistics in which space or physical matrices that assume data on a spatial grid are systematically considered. It involves analytical techniques adapted for data where observations have location information (either in two dimensions, i.e., longitude and latitude or in three dimensions - longitude, latitude and altitude) attached to them.
Spatial statistics allow for the modeling and understanding of the interaction between points in space, giving a much more profound insight for the data analyst or the geographer. It is usually applied to phenomena that show a pattern of spatial correlation and integrates different spatial aspects, testing or predicting real-world phenomena.
Common tools used in spatial statistics include spatial autocorrelation, spatial interpolation, geostatistics, point pattern analysis, and spatial regression. These techniques are used in diverse fields such as environmental science, health science, wildlife management, natural resource management, urban planning, landscape ecology, and socioeconomic studies.
Work faster with spatial data
Easily import data, automate analysis and build spatial apps for the web, all within a single software.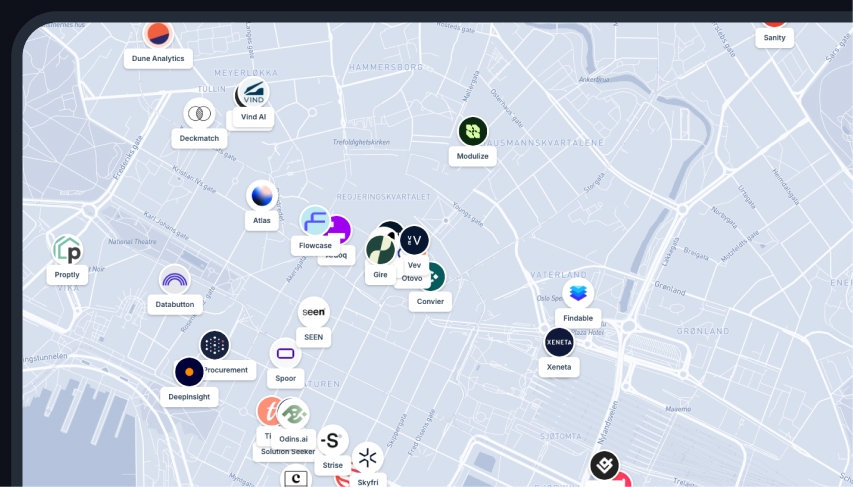
FAQs
What is the difference between spatial statistics and traditional statistics?
Spatial statistics differ from traditional statistics in that the former incorporate space (geography) into their methodology, accounting for spatial dependence and spatial heterogeneity, while the latter do not. The spatial location of each observation, as well as its relationship with other observations based on their geographical relationships, are considered in spatial statistics.
Why is Spatial Statistics important?
Spatial statistics is essential because almost all data are spatial in nature. Considering the spatial component of data helps in identifying patterns, modelling spatial relationships, predicting outcomes, and decision-making processes. Clear understanding of spatial patterns aids in resource allocation and policy implications.
What are some examples of Spatial Statistics applications?
Spatial statistics are used widely across various fields. In health sciences, for example, it's used to monitor disease spread over different geographical locations. In environmental sciences, spatial statistics can be used to assess the effect of climate change on species distribution. Other applications include crime mapping in criminology, evaluation of retail locations in business, and even in astronomy for galaxy distribution mapping.
What are common methods and tools used in Spatial Statistics?
Spatial autocorrelation, spatial interpolation, spatial regression, geostatistics and point pattern analysis are common methods used in spatial statistics. These techniques have been incorporated into various software for spatial data analytics such as Atlas.co, Python's geopandas, and R’s spdep and spatstat packages. These tools allow for the analysis and visualization of spatial data, and are widely used by spatial statisticians, geographers, and data scientists.