Geographic Weighted Regression
Definition
Geographic Weighted Regression (GWR) is an advanced statistical technique used in geographic information systems (GIS) to model spatially varying relationships. It extends traditional regression analysis by allowing the coefficients of the model to vary across space. This method recognizes that data collected across different geographical locations may not be uniformly distributed and that relationships between variables can change depending on spatial context. GWR is particularly valuable for analyzing spatially heterogenous data, providing more localized insights rather than a single global model.
What is Geographic Weighted Regression?
Geographic Weighted Regression is a local modeling technique employed within the domain of spatial analysis to explore and account for spatial non-stationarity. It provides a means to assess how the relationships between dependent and independent variables differ across locations. Unlike traditional regression that provides a single equation to describe the relationship between data variables, GWR generates location-specific models. This is achieved by incorporating a spatial weighting function that gives different weights to observations based on their geographical proximity to the location under consideration.
In practice, GWR calculates a separate set of regression coefficients for every location in a dataset, reflecting the local spatial characteristics. This is done using a moving window or kernel approach, where observations closer to a target location are given more influence than those further away. The flexibility and localized nature of GWR make it particularly useful in fields such as environmental science, urban planning, and public health, where spatial heterogeneity is often a key consideration.
Work faster with spatial data
Easily import data, automate analysis and build spatial apps for the web, all within a single software.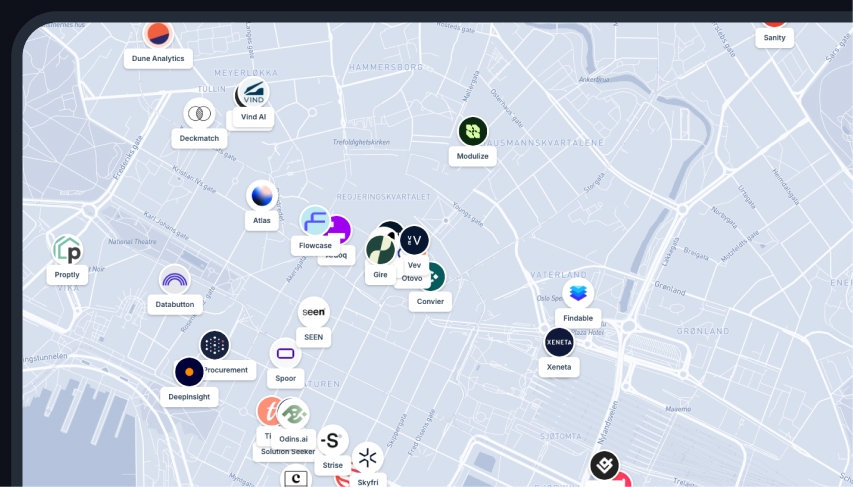
FAQs
What are the main uses of Geographic Weighted Regression?
GWR is mainly used in areas where understanding the spatial variation of relationships is crucial, such as environmental sciences, real estate, epidemiology, and urban planning. It helps in identifying and modeling spatial heterogeneity within datasets.
How does Geographic Weighted Regression differ from ordinary regression?
Unlike ordinary regression, which assumes a constant relationship across space, GWR accounts for spatial variability by allowing relationships to change from one location to another. Therefore, it generates location-specific coefficients instead of a single global coefficient set.
What are the limitations of Geographic Weighted Regression?
GWR can be computationally intensive due to the need to calculate multiple local models. There is also a risk of overfitting, as GWR can produce complex models that capture noise rather than true patterns, especially with sparse data.
What is spatial non-stationarity, and why is it important in GWR?
Spatial non-stationarity refers to the phenomenon where relationships between variables change across space. It is a crucial concept in GWR, as the method specifically addresses and models such spatial variability, providing insights that would be missed with traditional global models.