Bayesian GIS Analysis
Definition
Bayesian GIS Analysis is an approach within Geographic Information Systems (GIS) that applies Bayesian statistical methods for spatial data analysis. This methodology integrates the Bayesian framework, which involves updating the probability for a hypothesis as more evidence or information becomes available. It is particularly valuable in situations where data is uncertain or incomplete, allowing for more robust predictions and inferences about the spatial relationships and patterns that may not be apparent through traditional GIS analysis methods.
What is Bayesian GIS Analysis?
Bayesian GIS Analysis is an advanced analytical process used to interpret spatial data through the lens of Bayesian statistics. Unlike frequentist methods, Bayesian analysis incorporates prior knowledge or beliefs in addition to the data at hand, which is updated as new data becomes available. This results in a posterior distribution that reflects all available evidence.
In the context of GIS, Bayesian analysis is used to assess spatial data, making predictions about spatial phenomena, estimating unknown parameters, and updating these estimates as new spatial data is collected. This process involves defining a prior distribution for the parameters of interest, expressing beliefs about these parameters before any data is observed. Spatial data is then collected and used to form a likelihood function, describing the probability of observing the data given the parameters. Combining these two elements, the Bayesian framework calculates the posterior distribution, providing an updated belief about the parameter values after considering the data.
Bayesian GIS Analysis is particularly useful in areas such as environmental modeling, where predicting spatial patterns, such as species distributions or pollution levels, often involves uncertain data. It is also applied in fields such as epidemiology, for modeling the spread of diseases with spatial components. This analysis is valued for its ability to handle various sources of uncertainty and incorporate diverse data types, making it a powerful tool for spatial decision-making.
Work faster with spatial data
Easily import data, automate analysis and build spatial apps for the web, all within a single software.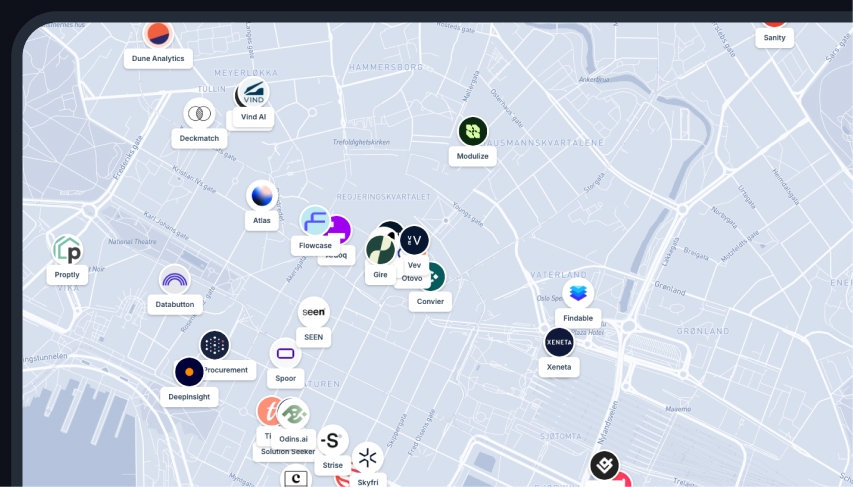
FAQs
What are the advantages of Bayesian GIS Analysis?
Bayesian GIS Analysis offers several advantages, including the ability to incorporate prior knowledge and beliefs, effectively handle uncertainty, and continuously update predictions with new data. It provides a more flexible and robust framework for spatial analysis compared to traditional methods.
How is Bayesian GIS Analysis applied in environmental modeling?
In environmental modeling, Bayesian GIS Analysis is used to predict spatial patterns, such as species distributions or pollution concentrations. It allows for the incorporation of prior environmental knowledge and handles various uncertainties in the data, making predictions more accurate and credible.
Does Bayesian GIS Analysis require specialized software?
Yes, performing Bayesian GIS Analysis typically requires specialized software that can handle complex statistical computations and spatial data. This software must be capable of implementing Bayesian statistical methods and managing large spatial datasets effectively.
What kind of data is needed for Bayesian GIS Analysis?
Bayesian GIS Analysis can be conducted with various types of spatial data, including raster and vector data, as well as non-spatial data that can influence spatial outcomes. The core requirement is that there must be some prior information or beliefs, along with data that can be used to update these beliefs.